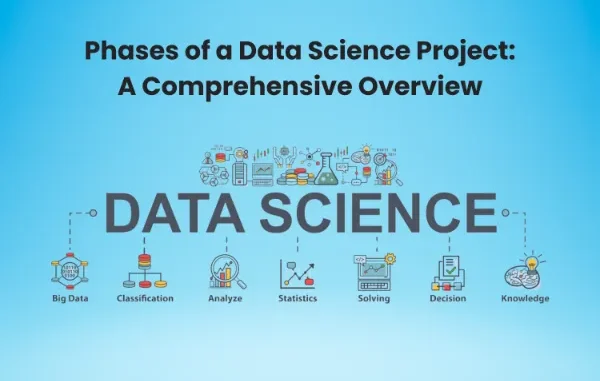
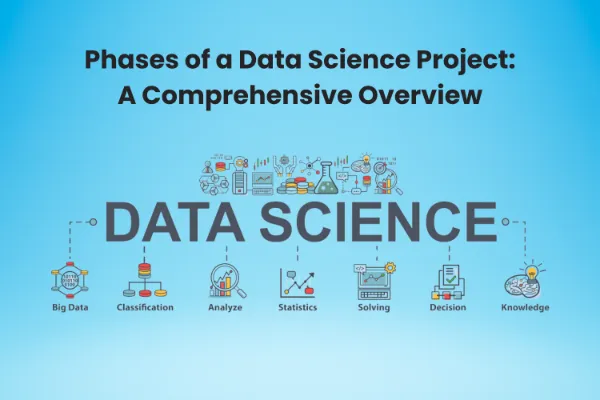
Starting a data science journey is like setting out on an unknown voyage with your analytical skills as the compass and the data set in front of you as the map. It is essential to understand the complexities of a data science project. Understanding the phases of a data science project is crucial to success, regardless of experience level in data science or data analytics.
In this blog, we dissect this procedure, exploring the subtleties of data modeling, deployment, and exploration. We’ll also discuss the value of Data Science Training Courses and how they fit in with the larger picture of the Data Science Life Cycle.
Table of Contents
- Data Exploration
- Data Preprocessing
- Feature Engineering
- Modelling
- Evaluation
- Deployment
- Interpretation
- Conclusion
Data Exploration
Any data science project’s beginning is like an artist with an empty canvas in front of them, full of possibilities. Analysts delve into the raw data during the data exploration stage to find patterns, anomalies, and maybe insightful information. This stage establishes the framework for the whole project as it entails figuring out how to handle outliers, discovering missing numbers, and comprehending the subtleties of the dataset. Here, data science training programs are essential because they provide professionals with the tools to sift through massive amounts of data and extract relevant information.
Data Preprocessing
Data preparation is the next natural step once the raw data has been carefully examined. Imagine it like polishing a gemstone’s rough edges to bring forth its actual brightness. This step ensures the data is compatible with the selected models, including cleaning the data, addressing missing values, and converting variables. This systematic procedure clarifies the importance of comprehending the data science life cycle. Data scientists must go from exploration to preprocessing easily, ensuring the data is ready for the following analysis steps.
Feature Engineering
The artifice of the approach shows as data scientists go through the feature engineering phase of the project. In feature engineering, characteristics that improve the model’s prediction ability are chosen, altered, and created. This stage is when analytical skills and domain knowledge often come together to shape the data into a nuanced palette for the modelling stage that follows. With their focus on practical applications, data science training programs enable professionals to skilfully handle the complexity of feature engineering.
Modelling
Now that the foundation has been established, it’s time to enter the modelling phase, which is the centre of a data science project. Here, data scientists choose their algorithms depending on the issue they are dealing with and the properties of the data. There is a clear interaction between theory and practice, and decisions taken at this stage greatly influence how the project turns out. The information gained from data science courses is crucial since it helps practitioners choose, adjust, and assess models to guarantee peak performance.
Evaluation
The assessment stage begins when the algorithms start to function, much like when you take a step back to appreciate a picture. Here, the models’ performance is carefully evaluated by contrasting expected and actual results. A quantitative assessment of the model’s effectiveness is provided by metrics like accuracy, precision, and recall, which take centre stage. Data scientists may modify and improve their analytical technique by gaining valuable insights into the strengths and limits of their approach via the assessment lens.
Deployment
The data science life cycle culminates in the deployment phase, allowing analytical labour results to be used in real-world scenarios. This stage entails incorporating the model into already-in-use systems, guaranteeing smooth operation, and assisting in the valuable implementation of insights. In this situation, experts with a comprehensive awareness of both analytical and operational needs negotiate the complexity of deployment with the help of skills refined via data science training courses.
Interpretation
The interpretation stage becomes the storyteller’s domain in the middle of data points and algorithms. Here, data scientists use the insights to create tales, turning raw data into stories that stakeholders can take action on. This stage is a fine art that calls for a sophisticated comprehension of the data and the environment in which it functions. Armed with the expertise from data science training courses, data scientists, like a professional novelist crafting a fascinating story, bring data to life and make sure it resonates meaningfully within the larger corporate environment.
Conclusion
Every step of a data science project contributes to developing practical insights, resulting in a well-balanced symphony. The trip is made more enjoyable by the ongoing learning that occurs naturally in data science training courses, from the first data exploration to the application of analytical models. Unlocking the full potential of data-driven decision-making requires recognising the entire nature of the data science life cycle as the digital ecosystem changes.
Leave a Reply