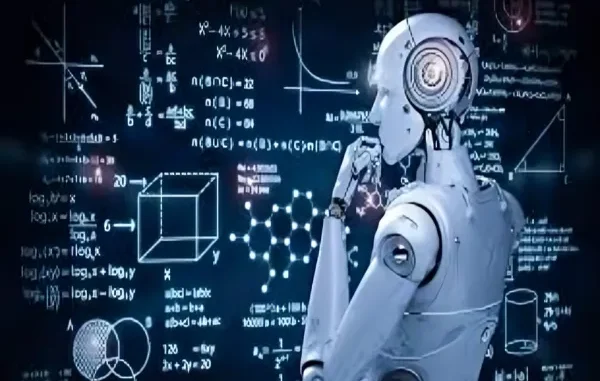
Introduction: The Intersection of Machine Learning and Investment
Machine learning (ML) is rapidly transforming various sectors, and finance is no exception. Its ability to analyze vast amounts of data and uncover patterns has revolutionized investment strategies. Investors and financial institutions are increasingly adopting ML to enhance decision-making, optimize strategies, and manage risks. In addition to insightful guidance, it provides a variety of educational resources to support investors in navigating complex financial landscapes. This article explores how ML is leveraged in investment, providing insights into its applications, benefits, and challenges.
Understanding Machine Learning in Finance
Machine learning refers to algorithms that enable computers to learn from and make predictions based on data. In finance, ML encompasses various techniques such as supervised learning, unsupervised learning, and reinforcement learning. Supervised learning involves training a model on labeled data, such as historical stock prices, to predict future trends. Unsupervised learning identifies hidden patterns in data without predefined labels, while reinforcement learning optimizes strategies through trial and error.
Predictive Analytics: Enhancing Forecast Accuracy
Predictive analytics uses historical data and machine learning algorithms to forecast future events. In finance, it is used to predict stock prices, market trends, and economic indicators. ML models like regression analysis and neural networks can process complex data and improve prediction accuracy. For instance, models can analyze patterns in trading volumes, price movements, and news sentiment to provide actionable insights. Successful examples include the use of deep learning models for high-frequency trading and market forecasting.
Algorithmic Trading: Revolutionizing Trade Execution

Algorithmic trading involves using algorithms to execute trades based on predefined criteria. Machine learning enhances algorithmic trading by analyzing market conditions and optimizing trade execution strategies. ML algorithms can process vast amounts of real-time data to make quick trading decisions, minimizing human intervention. Techniques such as quantitative trading, high-frequency trading, and sentiment-driven trading rely heavily on ML. These algorithms can adjust trading strategies in real time based on market dynamics, leading to more efficient and profitable trades.
Portfolio Management: Optimization Through Data
Machine learning plays a crucial role in portfolio management by optimizing asset allocation and risk management. ML models can analyze historical performance, market conditions, and economic indicators to construct and manage investment portfolios. Techniques such as mean-variance optimization and machine learning-based risk models help in diversifying investments and minimizing risks. For example, ML can identify correlations between assets and predict future performance, enabling better portfolio diversification and risk-adjusted returns.
Sentiment Analysis: Gauging Market Mood
Sentiment analysis involves interpreting and quantifying the sentiment expressed in textual data, such as news articles and social media posts. Machine learning techniques, particularly natural language processing (NLP), are used to analyze sentiment and gauge market mood. By analyzing sentiment trends, investors can assess market sentiment and make informed decisions. For example, NLP algorithms can evaluate the tone of financial news and social media chatter to predict market reactions and investment opportunities.
Fraud Detection and Prevention: Securing Investments
Fraud detection is a critical application of machine learning in finance. ML algorithms can identify unusual patterns and anomalies in transaction data, helping detect fraudulent activities. Techniques such as anomaly detection and pattern recognition are employed to monitor transactions and flag suspicious behavior. Machine learning models can learn from historical fraud data to improve detection accuracy and reduce false positives. For instance, credit card companies use ML to identify fraudulent transactions by analyzing spending patterns and transaction anomalies.
Challenges and Limitations of Machine Learning in Investing
Despite its advantages, machine learning in finance faces several challenges. Data quality is crucial, as ML models are only as good as the data they are trained on. Inaccurate or incomplete data can lead to erroneous predictions. Additionally, ML models can suffer from overfitting, where they perform well on training data but fail to generalize to new data. Transparency and interpretability of ML models are also concerns, as complex models can be difficult to understand and validate.
The Future of Machine Learning in Finance
The future of machine learning in finance is promising, with ongoing advancements and innovations. Emerging trends include the integration of artificial intelligence (AI) with ML for more sophisticated analyses and the use of big data for enhanced insights. Quantum computing may further revolutionize ML by processing complex calculations faster. Investors should stay abreast of these developments to leverage new technologies and maintain a competitive edge.
Conclusion: Embracing the Machine Learning Revolution
Machine learning is reshaping the investment landscape by providing advanced insights, optimizing strategies, and managing risks. Its applications in predictive analytics, algorithmic trading, portfolio management, sentiment analysis, and fraud detection offer significant advantages. However, challenges such as data quality and model interpretability must be addressed. As ML continues to evolve, it will play an increasingly vital role in shaping the future of finance. Investors who embrace these technologies will be better equipped to navigate the complexities of the financial markets.
Leave a Reply